Backpropagation¶
Course outline:¶
Backpropagation and chaine rule
Lab: with numpy and pytorch
%matplotlib inline
Backpropagation and chaine rule¶
We will set up a two layer network source pytorch tuto :
A fully-connected ReLU network with one hidden layer and no biases, trained to predict y from x using Euclidean error.
Chaine rule¶
Forward pass with local partial derivatives of ouput given inputs:
Backward: compute gradient of the loss given each parameters vectors applying chaine rule from the loss downstream to the parameters:
For \(w^{(2)}\):
For \(w^{(1)}\):
Recap: Vector derivatives¶
Given a function \(z = x\) with \(z\) the output, \(x\) the input and \(w\) the coeficients.
Scalar to Scalar: \(x \in \mathbb{R}, z \in \mathbb{R}\), \(w \in \mathbb{R}\)
Regular derivative:
If \(w\) changes by a small amount, how much will \(z\) change?
Vector to Scalar: \(x \in \mathbb{R}^N, z \in \mathbb{R}\), \(w \in \mathbb{R}^N\)
Derivative is Gradient of partial derivative: \(\frac{\partial z}{\partial w} \in \mathbb{R}^N\)
For each element \(w_i\) of \(w\), if it changes by a small amount then how much will y change?
Vector to Vector: \(w \in \mathbb{R}^N, z \in \mathbb{R}^M\)
Derivative is Jacobian of partial derivative:
TO COMPLETE
\(\frac{\partial z}{\partial w} \in \mathbb{R}^{N \times M}\)
Backpropagation summary¶
Backpropagation algorithm in a graph: 1. Forward pass, for each node compute local partial derivatives of ouput given inputs 2. Backward pass: apply chain rule from the end to each parameters - Update parameter with gradient descent using the current upstream gradient and the current local gradient - Compute upstream gradient for the backward nodes
Think locally and remember that at each node: - For the loss the gradient is the error - At each step, the upstream gradient is obtained by multiplying the upstream gradient (an error) with the current parameters (vector of matrix). - At each step, the current local gradient equal the input, therfore the current update is the current upstream gradient time the input.
import numpy as np
import matplotlib.pyplot as plt
import seaborn as sns
import sklearn.model_selection
Lab: with numpy and pytorch¶
Load iris data set¶
Goal: Predict Y = [petal_length, petal_width] = f(X = [sepal_length, sepal_width])
Plot data with seaborn
Remove setosa samples
Recode ‘versicolor’:1, ‘virginica’:2
Scale X and Y
Split data in train/test 50%/50%
iris = sns.load_dataset("iris")
#g = sns.pairplot(iris, hue="species")
df = iris[iris.species != "setosa"]
g = sns.pairplot(df, hue="species")
df['species_n'] = iris.species.map({'versicolor':1, 'virginica':2})
# Y = 'petal_length', 'petal_width'; X = 'sepal_length', 'sepal_width')
X_iris = np.asarray(df.loc[:, ['sepal_length', 'sepal_width']], dtype=np.float32)
Y_iris = np.asarray(df.loc[:, ['petal_length', 'petal_width']], dtype=np.float32)
label_iris = np.asarray(df.species_n, dtype=int)
# Scale
from sklearn.preprocessing import StandardScaler
scalerx, scalery = StandardScaler(), StandardScaler()
X_iris = scalerx.fit_transform(X_iris)
Y_iris = StandardScaler().fit_transform(Y_iris)
# Split train test
X_iris_tr, X_iris_val, Y_iris_tr, Y_iris_val, label_iris_tr, label_iris_val = \
sklearn.model_selection.train_test_split(X_iris, Y_iris, label_iris, train_size=0.5, stratify=label_iris)
/home/edouard/anaconda3/lib/python3.7/site-packages/ipykernel_launcher.py:5: SettingWithCopyWarning:
A value is trying to be set on a copy of a slice from a DataFrame.
Try using .loc[row_indexer,col_indexer] = value instead
See the caveats in the documentation: http://pandas.pydata.org/pandas-docs/stable/indexing.html#indexing-view-versus-copy
"""
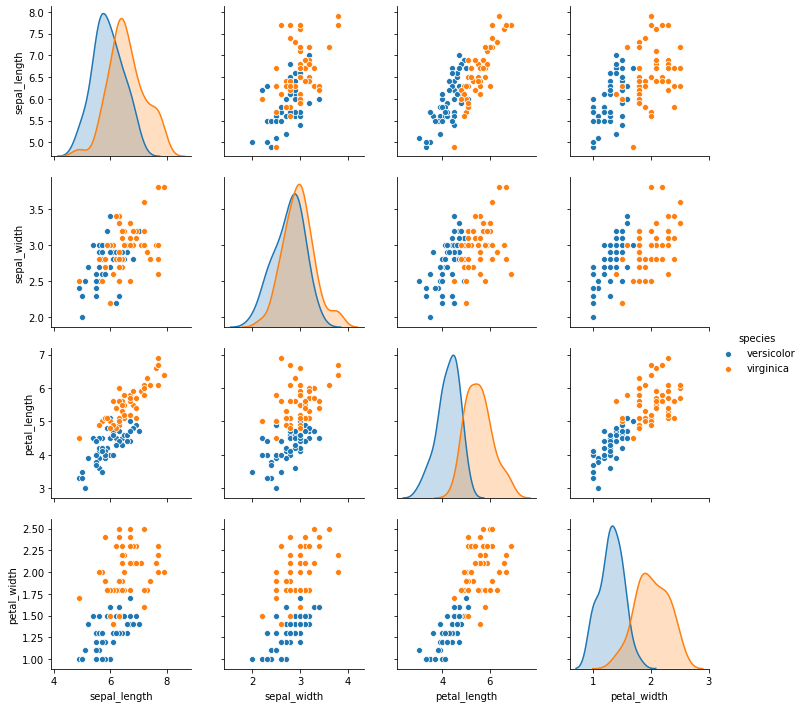
Backpropagation with numpy¶
This implementation uses numpy to manually compute the forward pass, loss, and backward pass.
# X=X_iris_tr; Y=Y_iris_tr; X_val=X_iris_val; Y_val=Y_iris_val
def two_layer_regression_numpy_train(X, Y, X_val, Y_val, lr, nite):
# N is batch size; D_in is input dimension;
# H is hidden dimension; D_out is output dimension.
# N, D_in, H, D_out = 64, 1000, 100, 10
N, D_in, H, D_out = X.shape[0], X.shape[1], 100, Y.shape[1]
W1 = np.random.randn(D_in, H)
W2 = np.random.randn(H, D_out)
losses_tr, losses_val = list(), list()
learning_rate = lr
for t in range(nite):
# Forward pass: compute predicted y
z1 = X.dot(W1)
h1 = np.maximum(z1, 0)
Y_pred = h1.dot(W2)
# Compute and print loss
loss = np.square(Y_pred - Y).sum()
# Backprop to compute gradients of w1 and w2 with respect to loss
grad_y_pred = 2.0 * (Y_pred - Y)
grad_w2 = h1.T.dot(grad_y_pred)
grad_h1 = grad_y_pred.dot(W2.T)
grad_z1 = grad_h1.copy()
grad_z1[z1 < 0] = 0
grad_w1 = X.T.dot(grad_z1)
# Update weights
W1 -= learning_rate * grad_w1
W2 -= learning_rate * grad_w2
# Forward pass for validation set: compute predicted y
z1 = X_val.dot(W1)
h1 = np.maximum(z1, 0)
y_pred_val = h1.dot(W2)
loss_val = np.square(y_pred_val - Y_val).sum()
losses_tr.append(loss)
losses_val.append(loss_val)
if t % 10 == 0:
print(t, loss, loss_val)
return W1, W2, losses_tr, losses_val
W1, W2, losses_tr, losses_val = two_layer_regression_numpy_train(X=X_iris_tr, Y=Y_iris_tr, X_val=X_iris_val, Y_val=Y_iris_val,
lr=1e-4, nite=50)
plt.plot(np.arange(len(losses_tr)), losses_tr, "-b", np.arange(len(losses_val)), losses_val, "-r")
0 15126.224825529907 2910.260853330454
10 71.5381374591153 104.97056197642135
20 50.756938353833334 80.02800827986354
30 46.546510744624236 72.85211241738614
40 44.41413064447564 69.31127324764276
[<matplotlib.lines.Line2D at 0x7f960cf5e9b0>,
<matplotlib.lines.Line2D at 0x7f960cf5eb00>]
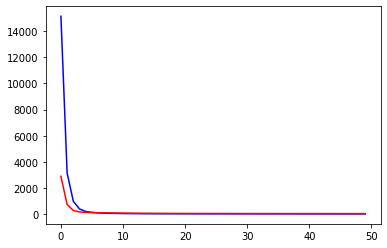
Backpropagation with PyTorch Tensors¶
Numpy is a great framework, but it cannot utilize GPUs to accelerate its numerical computations. For modern deep neural networks, GPUs often provide speedups of 50x or greater, so unfortunately numpy won’t be enough for modern deep learning. Here we introduce the most fundamental PyTorch concept: the Tensor. A PyTorch Tensor is conceptually identical to a numpy array: a Tensor is an n-dimensional array, and PyTorch provides many functions for operating on these Tensors. Behind the scenes, Tensors can keep track of a computational graph and gradients, but they’re also useful as a generic tool for scientific computing. Also unlike numpy, PyTorch Tensors can utilize GPUs to accelerate their numeric computations. To run a PyTorch Tensor on GPU, you simply need to cast it to a new datatype. Here we use PyTorch Tensors to fit a two-layer network to random data. Like the numpy example above we need to manually implement the forward and backward passes through the network:
import torch
# X=X_iris_tr; Y=Y_iris_tr; X_val=X_iris_val; Y_val=Y_iris_val
def two_layer_regression_tensor_train(X, Y, X_val, Y_val, lr, nite):
dtype = torch.float
device = torch.device("cpu")
# device = torch.device("cuda:0") # Uncomment this to run on GPU
# N is batch size; D_in is input dimension;
# H is hidden dimension; D_out is output dimension.
N, D_in, H, D_out = X.shape[0], X.shape[1], 100, Y.shape[1]
# Create random input and output data
X = torch.from_numpy(X)
Y = torch.from_numpy(Y)
X_val = torch.from_numpy(X_val)
Y_val = torch.from_numpy(Y_val)
# Randomly initialize weights
W1 = torch.randn(D_in, H, device=device, dtype=dtype)
W2 = torch.randn(H, D_out, device=device, dtype=dtype)
losses_tr, losses_val = list(), list()
learning_rate = lr
for t in range(nite):
# Forward pass: compute predicted y
z1 = X.mm(W1)
h1 = z1.clamp(min=0)
y_pred = h1.mm(W2)
# Compute and print loss
loss = (y_pred - Y).pow(2).sum().item()
# Backprop to compute gradients of w1 and w2 with respect to loss
grad_y_pred = 2.0 * (y_pred - Y)
grad_w2 = h1.t().mm(grad_y_pred)
grad_h1 = grad_y_pred.mm(W2.t())
grad_z1 = grad_h1.clone()
grad_z1[z1 < 0] = 0
grad_w1 = X.t().mm(grad_z1)
# Update weights using gradient descent
W1 -= learning_rate * grad_w1
W2 -= learning_rate * grad_w2
# Forward pass for validation set: compute predicted y
z1 = X_val.mm(W1)
h1 = z1.clamp(min=0)
y_pred_val = h1.mm(W2)
loss_val = (y_pred_val - Y_val).pow(2).sum().item()
losses_tr.append(loss)
losses_val.append(loss_val)
if t % 10 == 0:
print(t, loss, loss_val)
return W1, W2, losses_tr, losses_val
W1, W2, losses_tr, losses_val = two_layer_regression_tensor_train(X=X_iris_tr, Y=Y_iris_tr, X_val=X_iris_val, Y_val=Y_iris_val,
lr=1e-4, nite=50)
plt.plot(np.arange(len(losses_tr)), losses_tr, "-b", np.arange(len(losses_val)), losses_val, "-r")
0 8086.1591796875 5429.57275390625
10 225.77589416503906 331.83734130859375
20 86.46501159667969 117.72447204589844
30 52.375606536865234 73.84156036376953
40 43.16458511352539 64.0667495727539
[<matplotlib.lines.Line2D at 0x7f960033c470>,
<matplotlib.lines.Line2D at 0x7f960033c5c0>]
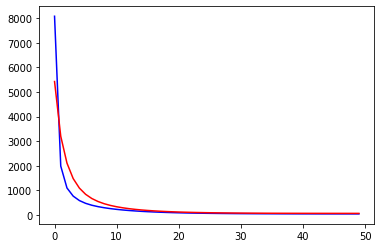
Backpropagation with PyTorch: Tensors and autograd¶
A fully-connected ReLU network with one hidden layer and no biases,
trained to predict y from x by minimizing squared Euclidean distance.
This implementation computes the forward pass using operations on
PyTorch Tensors, and uses PyTorch autograd to compute gradients. A
PyTorch Tensor represents a node in a computational graph. If x
is a
Tensor that has x.requires_grad=True
then x.grad
is another
Tensor holding the gradient of x
with respect to some scalar value.
import torch
# X=X_iris_tr; Y=Y_iris_tr; X_val=X_iris_val; Y_val=Y_iris_val
# del X, Y, X_val, Y_val
def two_layer_regression_autograd_train(X, Y, X_val, Y_val, lr, nite):
dtype = torch.float
device = torch.device("cpu")
# device = torch.device("cuda:0") # Uncomment this to run on GPU
# N is batch size; D_in is input dimension;
# H is hidden dimension; D_out is output dimension.
N, D_in, H, D_out = X.shape[0], X.shape[1], 100, Y.shape[1]
# Setting requires_grad=False indicates that we do not need to compute gradients
# with respect to these Tensors during the backward pass.
X = torch.from_numpy(X)
Y = torch.from_numpy(Y)
X_val = torch.from_numpy(X_val)
Y_val = torch.from_numpy(Y_val)
# Create random Tensors for weights.
# Setting requires_grad=True indicates that we want to compute gradients with
# respect to these Tensors during the backward pass.
W1 = torch.randn(D_in, H, device=device, dtype=dtype, requires_grad=True)
W2 = torch.randn(H, D_out, device=device, dtype=dtype, requires_grad=True)
losses_tr, losses_val = list(), list()
learning_rate = lr
for t in range(nite):
# Forward pass: compute predicted y using operations on Tensors; these
# are exactly the same operations we used to compute the forward pass using
# Tensors, but we do not need to keep references to intermediate values since
# we are not implementing the backward pass by hand.
y_pred = X.mm(W1).clamp(min=0).mm(W2)
# Compute and print loss using operations on Tensors.
# Now loss is a Tensor of shape (1,)
# loss.item() gets the scalar value held in the loss.
loss = (y_pred - Y).pow(2).sum()
# Use autograd to compute the backward pass. This call will compute the
# gradient of loss with respect to all Tensors with requires_grad=True.
# After this call w1.grad and w2.grad will be Tensors holding the gradient
# of the loss with respect to w1 and w2 respectively.
loss.backward()
# Manually update weights using gradient descent. Wrap in torch.no_grad()
# because weights have requires_grad=True, but we don't need to track this
# in autograd.
# An alternative way is to operate on weight.data and weight.grad.data.
# Recall that tensor.data gives a tensor that shares the storage with
# tensor, but doesn't track history.
# You can also use torch.optim.SGD to achieve this.
with torch.no_grad():
W1 -= learning_rate * W1.grad
W2 -= learning_rate * W2.grad
# Manually zero the gradients after updating weights
W1.grad.zero_()
W2.grad.zero_()
y_pred = X_val.mm(W1).clamp(min=0).mm(W2)
# Compute and print loss using operations on Tensors.
# Now loss is a Tensor of shape (1,)
# loss.item() gets the scalar value held in the loss.
loss_val = (y_pred - Y).pow(2).sum()
if t % 10 == 0:
print(t, loss.item(), loss_val.item())
losses_tr.append(loss.item())
losses_val.append(loss_val.item())
return W1, W2, losses_tr, losses_val
W1, W2, losses_tr, losses_val = two_layer_regression_autograd_train(X=X_iris_tr, Y=Y_iris_tr, X_val=X_iris_val, Y_val=Y_iris_val,
lr=1e-4, nite=50)
plt.plot(np.arange(len(losses_tr)), losses_tr, "-b", np.arange(len(losses_val)), losses_val, "-r")
0 8307.1806640625 2357.994873046875
10 111.97289276123047 250.04209899902344
20 65.83244323730469 201.63694763183594
30 53.70908737182617 183.17051696777344
40 48.719329833984375 173.3616943359375
[<matplotlib.lines.Line2D at 0x7f95ff2ad978>,
<matplotlib.lines.Line2D at 0x7f95ff2adac8>]
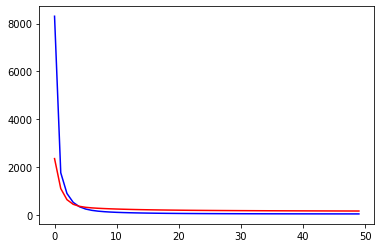
Backpropagation with PyTorch: nn¶
This implementation uses the nn package from PyTorch to build the network. PyTorch autograd makes it easy to define computational graphs and take gradients, but raw autograd can be a bit too low-level for defining complex neural networks; this is where the nn package can help. The nn package defines a set of Modules, which you can think of as a neural network layer that has produces output from input and may have some trainable weights.
import torch
# X=X_iris_tr; Y=Y_iris_tr; X_val=X_iris_val; Y_val=Y_iris_val
# del X, Y, X_val, Y_val
def two_layer_regression_nn_train(X, Y, X_val, Y_val, lr, nite):
# N is batch size; D_in is input dimension;
# H is hidden dimension; D_out is output dimension.
N, D_in, H, D_out = X.shape[0], X.shape[1], 100, Y.shape[1]
X = torch.from_numpy(X)
Y = torch.from_numpy(Y)
X_val = torch.from_numpy(X_val)
Y_val = torch.from_numpy(Y_val)
# Use the nn package to define our model as a sequence of layers. nn.Sequential
# is a Module which contains other Modules, and applies them in sequence to
# produce its output. Each Linear Module computes output from input using a
# linear function, and holds internal Tensors for its weight and bias.
model = torch.nn.Sequential(
torch.nn.Linear(D_in, H),
torch.nn.ReLU(),
torch.nn.Linear(H, D_out),
)
# The nn package also contains definitions of popular loss functions; in this
# case we will use Mean Squared Error (MSE) as our loss function.
loss_fn = torch.nn.MSELoss(reduction='sum')
losses_tr, losses_val = list(), list()
learning_rate = lr
for t in range(nite):
# Forward pass: compute predicted y by passing x to the model. Module objects
# override the __call__ operator so you can call them like functions. When
# doing so you pass a Tensor of input data to the Module and it produces
# a Tensor of output data.
y_pred = model(X)
# Compute and print loss. We pass Tensors containing the predicted and true
# values of y, and the loss function returns a Tensor containing the
# loss.
loss = loss_fn(y_pred, Y)
# Zero the gradients before running the backward pass.
model.zero_grad()
# Backward pass: compute gradient of the loss with respect to all the learnable
# parameters of the model. Internally, the parameters of each Module are stored
# in Tensors with requires_grad=True, so this call will compute gradients for
# all learnable parameters in the model.
loss.backward()
# Update the weights using gradient descent. Each parameter is a Tensor, so
# we can access its gradients like we did before.
with torch.no_grad():
for param in model.parameters():
param -= learning_rate * param.grad
y_pred = model(X_val)
loss_val = (y_pred - Y_val).pow(2).sum()
if t % 10 == 0:
print(t, loss.item(), loss_val.item())
losses_tr.append(loss.item())
losses_val.append(loss_val.item())
return model, losses_tr, losses_val
model, losses_tr, losses_val = two_layer_regression_nn_train(X=X_iris_tr, Y=Y_iris_tr, X_val=X_iris_val, Y_val=Y_iris_val,
lr=1e-4, nite=50)
plt.plot(np.arange(len(losses_tr)), losses_tr, "-b", np.arange(len(losses_val)), losses_val, "-r")
0 82.32025146484375 91.3389892578125
10 50.322200775146484 63.563087463378906
20 40.825225830078125 57.13555145263672
30 37.53572082519531 55.74506378173828
40 36.191200256347656 55.499732971191406
[<matplotlib.lines.Line2D at 0x7f95ff296668>,
<matplotlib.lines.Line2D at 0x7f95ff2967b8>]
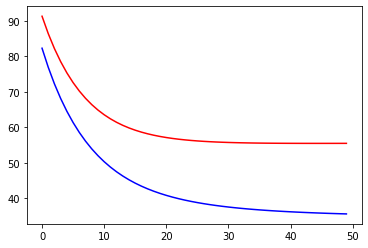
Backpropagation with PyTorch optim¶
This implementation uses the nn package from PyTorch to build the network. Rather than manually updating the weights of the model as we have been doing, we use the optim package to define an Optimizer that will update the weights for us. The optim package defines many optimization algorithms that are commonly used for deep learning, including SGD+momentum, RMSProp, Adam, etc.
import torch
# X=X_iris_tr; Y=Y_iris_tr; X_val=X_iris_val; Y_val=Y_iris_val
def two_layer_regression_nn_optim_train(X, Y, X_val, Y_val, lr, nite):
# N is batch size; D_in is input dimension;
# H is hidden dimension; D_out is output dimension.
N, D_in, H, D_out = X.shape[0], X.shape[1], 100, Y.shape[1]
X = torch.from_numpy(X)
Y = torch.from_numpy(Y)
X_val = torch.from_numpy(X_val)
Y_val = torch.from_numpy(Y_val)
# Use the nn package to define our model and loss function.
model = torch.nn.Sequential(
torch.nn.Linear(D_in, H),
torch.nn.ReLU(),
torch.nn.Linear(H, D_out),
)
loss_fn = torch.nn.MSELoss(reduction='sum')
losses_tr, losses_val = list(), list()
# Use the optim package to define an Optimizer that will update the weights of
# the model for us. Here we will use Adam; the optim package contains many other
# optimization algoriths. The first argument to the Adam constructor tells the
# optimizer which Tensors it should update.
learning_rate = lr
optimizer = torch.optim.Adam(model.parameters(), lr=learning_rate)
for t in range(nite):
# Forward pass: compute predicted y by passing x to the model.
y_pred = model(X)
# Compute and print loss.
loss = loss_fn(y_pred, Y)
# Before the backward pass, use the optimizer object to zero all of the
# gradients for the variables it will update (which are the learnable
# weights of the model). This is because by default, gradients are
# accumulated in buffers( i.e, not overwritten) whenever .backward()
# is called. Checkout docs of torch.autograd.backward for more details.
optimizer.zero_grad()
# Backward pass: compute gradient of the loss with respect to model
# parameters
loss.backward()
# Calling the step function on an Optimizer makes an update to its
# parameters
optimizer.step()
with torch.no_grad():
y_pred = model(X_val)
loss_val = loss_fn(y_pred, Y_val)
if t % 10 == 0:
print(t, loss.item(), loss_val.item())
losses_tr.append(loss.item())
losses_val.append(loss_val.item())
return model, losses_tr, losses_val
model, losses_tr, losses_val = two_layer_regression_nn_optim_train(X=X_iris_tr, Y=Y_iris_tr, X_val=X_iris_val, Y_val=Y_iris_val,
lr=1e-3, nite=50)
plt.plot(np.arange(len(losses_tr)), losses_tr, "-b", np.arange(len(losses_val)), losses_val, "-r")
0 92.271240234375 83.96189880371094
10 64.25907135009766 59.872535705566406
20 47.6252555847168 50.228126525878906
30 40.33802032470703 50.60377502441406
40 38.19448471069336 54.03163528442383
[<matplotlib.lines.Line2D at 0x7f95ff200080>,
<matplotlib.lines.Line2D at 0x7f95ff2001d0>]
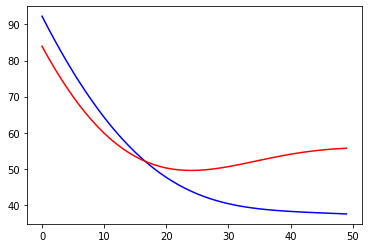